About Me
I am Xinyu Chen (陈新宇), a Postdoctoral Associate at MIT, working with Prof. Jinhua Zhao on data-driven machine learning challenges in computational engineering. I am currently involved in the Mens, Manus, and Machina (M3S) and Department of Energy (DOE) projects. My research focuses on developing theoretical and interpretable machine learning methods for modeling spatiotemporal data and computational social science data. The model development from a machine learning perspective can be summarized as tensor decomposition for machine learning (Tensor4ML) and optimization for interpretable machine learning (Opt4ML). In practice, the spatiotemporal datasets are often multidimensional tensors, including human mobility, trajectory data, traffic flow, fluid flow, climate/weather variable data, energy consumption, and international trade data. Our work addresses key scientific problems in computational engineering such as:
-
Spatiotemporal data imputation & prediction: Imputing and forecasting spatiotemporal data in the presence of missing values.
• Bayesian temporal factorization for multidimensional time series prediction (TPAMI 2022)
• Forecasting urban traffic states with sparse data using Hankel temporal matrix factorization (IJOC 2024)
• Forecasting sparse movement speed of urban road networks with nonstationary temporal matrix factorization (TS 2025)
-
Speed field reconstruction of traffic flow: Generating vehicular speed fields from partially observed trajectory data.
• Laplacian convolutional representation for traffic time series imputation (TKDE 2024)
-
Spatiotemporal pattern discovery: Discovering dynamic patterns from urban human mobility and climate systems.
• Discovering dynamic patterns from spatiotemporal data with time-varying low-rank autoregression (TKDE 2024)
• Dynamic autoregressive tensor factorization for pattern discovery of spatiotemporal systems
-
Time series periodicity quantification: Quantifying periodicity and seasonality of urban human mobility (i.e., daily/weekly regularity) and climate systems (i.e., yearly seasonality).
• Correlating time series with interpretable convolutional kernels (TKDE 2025)
• Interpretable time series autoregression
-
CS journals: IEEE Transactions on Pattern Analysis and Machine Intelligence (TPAMI), IEEE Transactions on Knowledge and Data Engineering (TKDE).
-
OR/MS journals: INFORMS Journal on Computing (IJOC), Transportation Science (TS).
-
Time Series Modeling: Time series convolution (100%)
-
LaTeX visualization: Awesome LaTeX drawing (4%)
Selected News
(For the full list of news, please check the News tab.)
-
March 2025: Our paper "Correlating time series with interpretable convolutional kernels" (authors: Xinyu Chen, HanQin Cai, Fuqiang Liu, Jinhua Zhao) was accepted to IEEE Transactions on Knowledge and Data Engineering.
-
February 2025: Our paper "Forecasting sparse movement speed of urban road networks with nonstationary temporal matrix factorization" (authors: Xinyu Chen, Chengyuan Zhang, Xi-Le Zhao, Nicolas Saunier, Lijun Sun) was accepted to Transportation Science.
-
August 2024: Our paper "Forecasting urban traffic states with sparse data using Hankel temporal matrix factorization" (authors: Xinyu Chen, Xi-Le Zhao, Chun Cheng) was accepted to INFORMS Journal on Computing.
-
June 2024: Our paper "Laplacian convolutional representation for traffic time series imputation" (authors: Xinyu Chen, Zhanhong Cheng, HanQin Cai, Nicolas Saunier, Lijun Sun) was accepted to IEEE Transactions on Knowledge and Data Engineering.
-
December 2023: I successfully passed my PhD research defense. It would be my great pleasure to have Prof. Francesco Ciari, Prof. Nicolas Saunier, Prof. Lijun Sun, Prof. James Goulet, and Prof. Guillaume Rabusseau as the jury members. If you are interested in my PhD research, welcome to take a look at the slides. Special thanks to my supervisor Prof. Nicolas Saunier for the great suggestion when I was organizing all materials.
-
July 2023: Our paper "Discovering dynamic patterns from spatiotemporal data with time-varying low-rank autoregression" (authors: Xinyu Chen, Chengyuan Zhang, Xiaoxu Chen, Nicolas Saunier, Lijun Sun) was accepted to IEEE Transactions on Knowledge and Data Engineering.
-
December 2021: I am delighted to be awarded the CIRRELT PhD Excellence Scholarship. For more details on Interuniversity Research Centre on Enterprise Networks, Logistics and Transportation (CIRRELT) and its mission, please check out the website.
-
March 2021: Our paper "Bayesian temporal factorization for multidimensional time series prediction" (authors: Xinyu Chen, Lijun Sun) was accepted to IEEE Transactions on Pattern Analysis and Machine Intelligence.
-
April 2020: I am delighted to be awarded the IVADO PhD Excellence Scholarship. The awarded research topic is "City-Scale Traffic Data Imputation and Forecasting with Tensor Learning". For more details on Institute for Data Valorisation (IVADO) and its mission, please check out the website.
Archive
vistors & views since September 2021
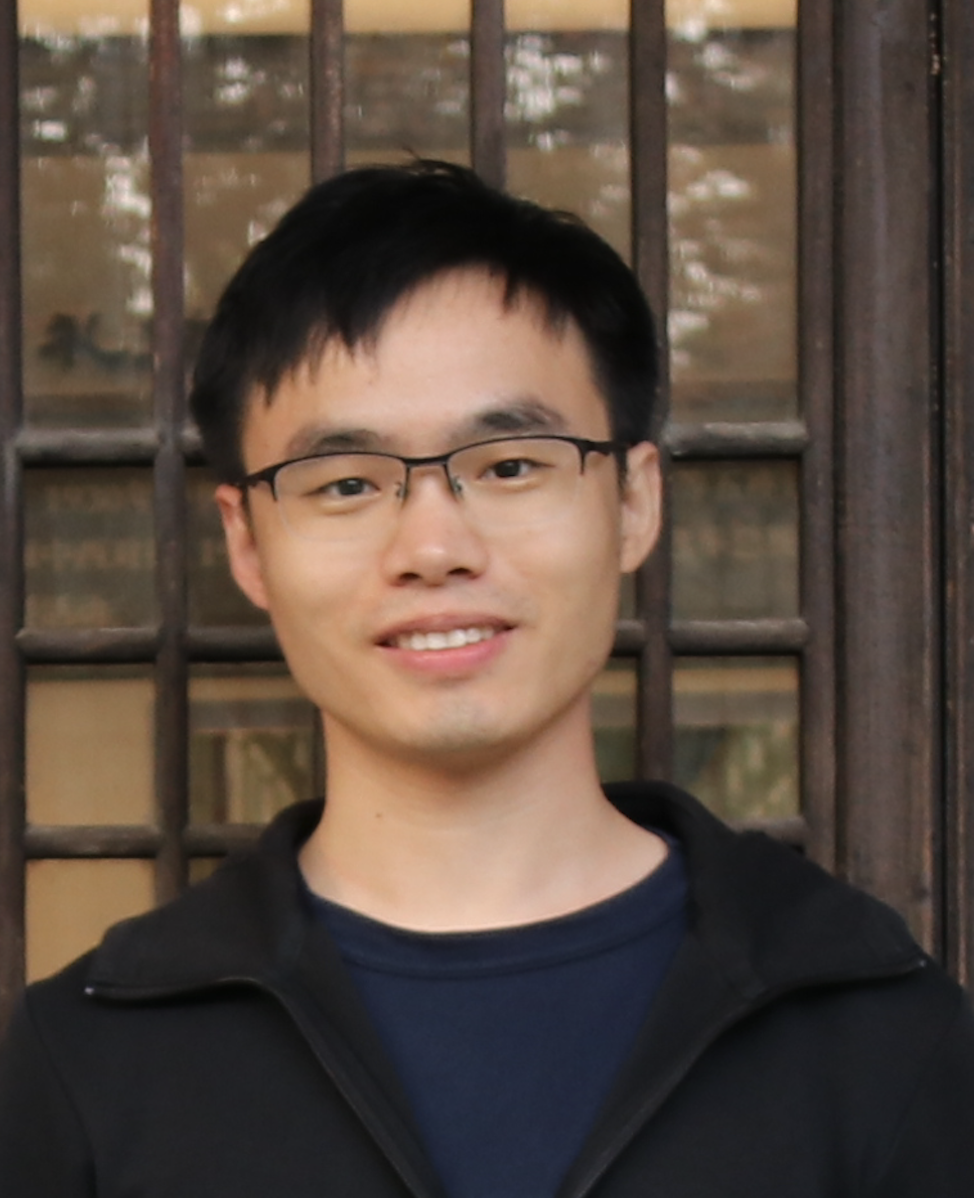
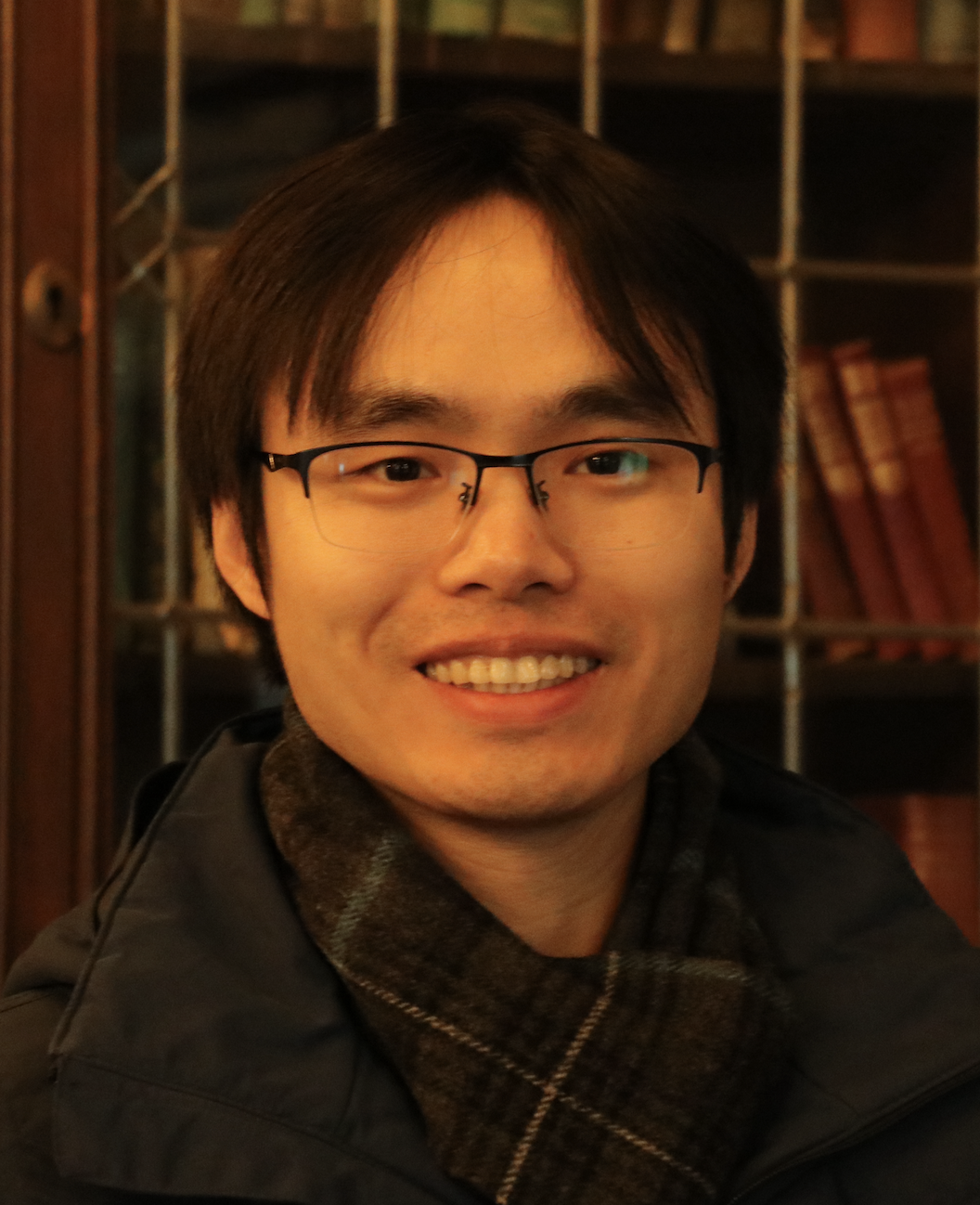
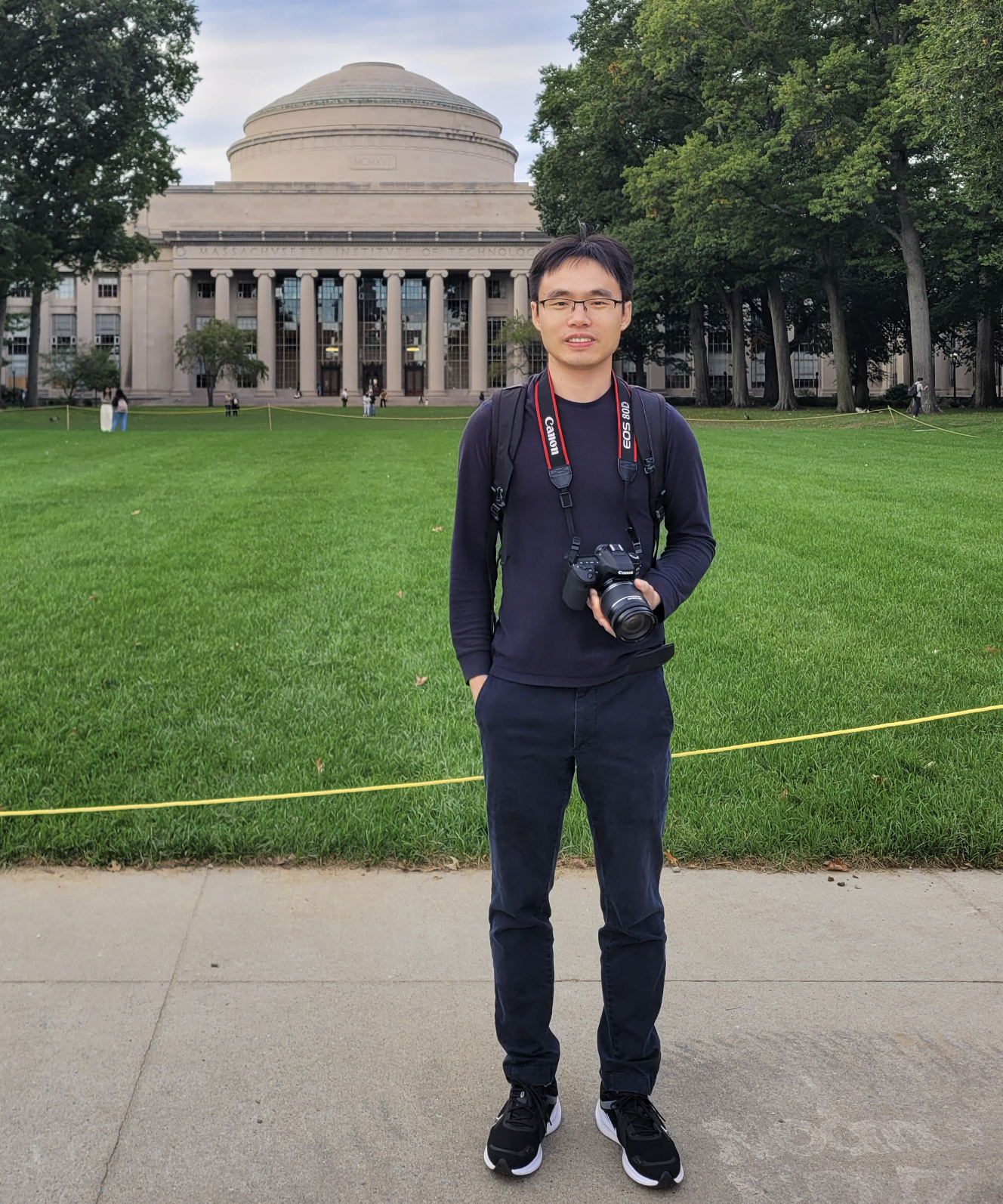